According to the 2020 World Health Organization (WHO) global Tuberculosis (TB) report, the Philippines has the highest TB incidence in Asia, with 554 cases for every 100 000 Filipinos. The Filipino response towards TB is exacerbated by the complex geography of the country - an archipelago of over 7100 islands - making the locating of TB cases particularly tricky. The result is that 31% of cases go undetected.
To address these challenges, EPCON, working in collaboration with the WHO and National Department of Health , implemented its platform, combining Artificial Intelligence and Machine Learning components, to estimate TB burden at subnational level. The project ran over a five month period in 2021/2022, evaluating national, provincial, municipality and barangay population clusters. A barangay is the smallest administrative division in the Philippines and is the native Filipino term for a village, district, or ward.
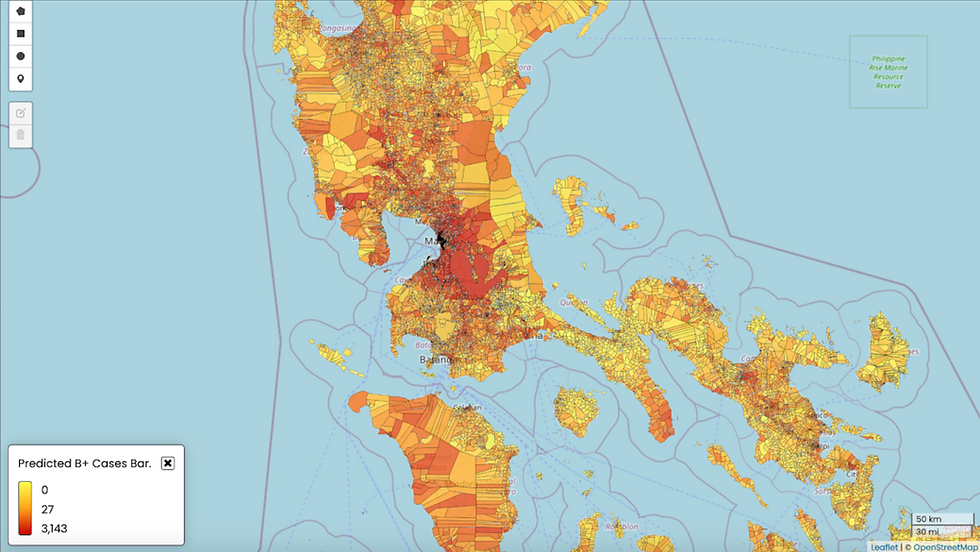
Working with limited data
Community screening data, which shows the ratio of positive to negative TB cases, is an essential statistic for modelling the prevalence of TB. However, at the onset of the project EPCON had access to a limited set of community screening data, comprising only 130 locations, mostly in the Northern areas, including Luzon, the country’s largest and most populous island. Applying EPCONs method, the screening data was combined with a variety of contextual data within the 42 000 barangays to assemble a digital representation of the real world for the AI model.
The contextual variables, which may have an effect on the individual’s TB exposure, included the following:
Socio-demographic data: population demographics, age structure, population density and vulnerable groups (including homeless, indigenous people and family in need of special protection at barangay level)
Indicators of disease, access to health care & resources: poverty, vaccination coverage, access to safe water and sanitation services, health facility coverage and accessibility to healthcare
Spatial-temporal environmental data: elevation, nightlights, slope, distance to major road intersections and waterways, and climate
Finding pockets of TB
The latest national prevalence survey in the Philippines, conducted in 2016, estimated the country's prevalence at around 1.2 million bacteriologically confirmed positive cases. EPCON’s AI platform, using the limited training set in combination with its high resolution contextual data, follows this trend. However, the EPCON model provides insights as to where the missing cases are likely to be found and who is mostly at risk.
As part of its output, a geographical data portal and dashboards were then introduced. The portal and dashboards allow the program team to evaluate the burden of TB on national, provincial, municipality or barangay, or even smaller arbitrary population clusters of suitable sizes. These projections help anticipate where communities are not receiving sufficient access to health care, and allow for prioritization of resources in function of risk, capacity or desired outcome.
Where to now
As part of its roll-out strategy, EPCON is currently validating the predictive model and further improving its accuracy. In addition, the team is engaging with in-country stakeholders for integrating the platform within the ongoing TB programs. The platform’s dynamic nature and output allow for a coordinated response and planning of tailored interventions. Our ambition is be to build dashboards for the national TB coordination team to provide a high-level aggregated view, and a more granular, disaggregated views to first line support healthcare partners.
The integration of programmatic data creates a closed loop and enables the incremental learning of the model, improving accuracy and allowing for real time monitoring and evaluation. By harnessing an AI predictive model in the fight against TB, the Philippines has opened a new vista for preventative care in public health - an approach likely to reap high rewards.